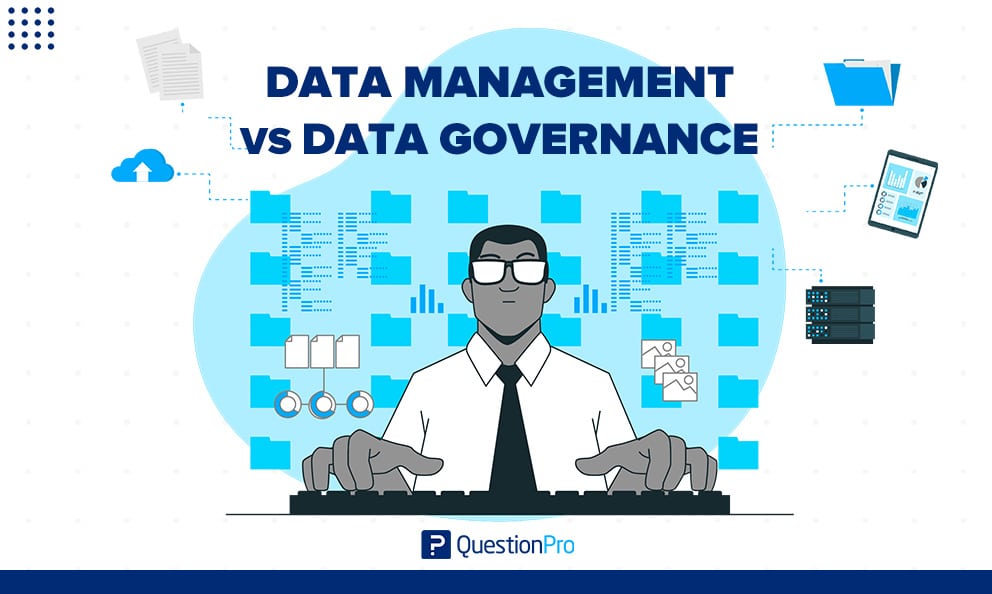
Data could be one of the most valuable parts of any business or organization. Today’s successful companies are defined by their ability to effectively analyze, use, and strategize with their company’s data. Poor data policies pose serious risks that could even harm an organization. To ensure that data is handled correctly, it’s essential to set up and understand data management vs data governance practices.
Big data allows firms to use enterprise data to obtain insights and make better decisions. Data management vs data governance offers solutions. With the help of this post, you will learn the fundamental differences between these functions by examining their definitions in detail.
What is data governance?
Data governance focuses on the policies, practices, and standards that comprise a company’s overall plan for properly processing and storing data. It assists the organization in strategic decision-making. It is an important business program that needs a policy, which is best reached by getting everyone in the company to agree.
A good data governance strategy is needed to ensure that an organization follows the rules, reduces risks, improves data security, and provides that someone is responsible for its data. To be successful, all of the essential parts of data governance must work together. Let’s have a look at some of the factors involved:
People
Nearly every employee uses data in some way or another. So, people are an essential part of data governance policies since they are the ones who create and manage the data and will ultimately benefit from well-governed data. Subject matter experts can define standardized business terms and quality thresholds for diverse business processes.
IT professionals and database administrators handle all aspects of technology. Data stewards address quality issues. Experts in law and security protect data privacy. The board or council of cross-functional executives is responsible for resolving organizational problems.
Policies and rules
Consent, quality, retention, and security are just a few of organizations’ policies and regulations to govern their operations and procedures. For example, a policy can specify who has access to and leverages the information, how long it is kept on file, and where specific sorts of data are kept.
You may have a policy that requires consent to use personal information. One rule may specify what forms of consent consumers must give when their personal information is acquired. Another rule states that a customer’s permission to be marketed needs to be verified before a promotional offer is sent to them.
Metrics
Technical metrics often include things like the number of duplicate records in an application. These also include how accurate and complete the data is and how many personal data elements are encrypted or hidden.
Financial analysts and lenders use days sales outstanding (DSO) to measure a company’s financial health. If DSO is above average, analysts and lenders may reduce the company’s forecast or raise the cost of financing. Incomplete client address data increases billing cycle time and DSO.
LEARN ABOUT: Data Management Framework
What is data management?
Data management is mostly about placing the strategy for data governance into action. It is the process of making and trying to implement the architectures, policies, and procedures that an organization needs to manage the full data workflow.
Using a data management strategy helps ensure that data is managed according to the overall company guidelines throughout its complete process, from when it is created until it is withdrawn.
Before we dive into the important differences between data governance and data management, let’s take a quick look back at the essential components of data management:
- Data preparation: Data preparation refers to the act of cleaning and modifying raw data so that it may be accurately examined. The completion of this process could take a considerable period of time. In the haste to complete the reporting and analysis, there is a risk that this essential first step may be skipped. As a result, businesses may end up making poor decisions based on inaccurate data.
- Pipelines of data: The process of automatically moving data from one system to another can be completed with the assistance of data pipelines. This goal is reached in a timely manner.
- Extract, convert, and load data into a database: This means making changes to data so it can be loaded into a business’s database system. Once they’ve been built, these are often done through automated processes. But usually, preparation and work on the pipeline are needed first.
- Catalogs of data: Organizing the data and making it easier to find and maintain track of it helps build a complete representation of the data.
- Data storage facilities: It makes the data analysis process significantly less challenging to carry out since they centralize all of the various data sources in one spot.
- Data governance: This can assist in defining the rules and procedures that need to be followed to maintain data compliance and security.
- The structure of data is the formally organized framework used to manage data flow.
- Safety of data: The components that make up your data security system are the safeguards that have been put in place to prevent your data from being altered or seen by individuals who are not allowed to do so.
Data governance vs. data management: The difference
Let’s take a closer look at data governance versus data management now. When it comes to implementing a data management plan, data governance provides the necessary direction.
Data Governance | Data Management |
This involves policies, regulations, and procedures to manage data quality. | This refers to how the data is managed. |
Refers to the application of knowledge, the development of procedures, and the formulation of theories. | Refers to the collection, organization, protection, processing, sorting, and preservation of data. |
It aims to ensure the accuracy and integrity of stored data. | It aims to improve the overall quality and monetary value. |
It’s a data strategy for obtaining high-quality data. | It’s a method for organizing facts in a logical way. |
Philosophical and business-focused. | Logistical and tech-focused. |
How do data governance vs data management interact?
Data governance and data management influence an organization’s capacity to accomplish its security and maintenance goals, with the former focusing on policies and the latter on procedures.
For example, a data governance policy may demand seven years of consumer data storage to comply with regulations. Then, data management operations with data management software can archive and delete data in storage systems.
Data governance and data management lifecycle are closely related concepts that work together to ensure that an organization’s data is effectively managed, protected, and used in a responsible and compliant manner. While they have distinct roles and objectives, they interact in several ways to support overall data quality, security, and compliance. Here’s how they interact:
Data governance sets the framework
Data governance is the overarching framework that defines the policies, procedures, and rules for managing data within an organization. It establishes the guidelines for data management practices. Data governance provides the structure and accountability needed to ensure that data is treated as a valuable data assets.
Data management implements governance
Data management is the practical implementation of data governance framework principles. It involves the processes, tools, and techniques used to collect, store, organize, and maintain data. Data management activities, such as data integration, data cleansing, and data storage, should align with the policies and rules set by data governance tools.
Data governance enforces compliance
Data governance program ensures that data management activities are carried out in compliance with relevant regulations and organizational policies. It sets the standards for data quality, data privacy, and data security, and effective data management teams must adhere to these standards while performing their tasks.
Data management feeds into governance
Data management processes and provides insights and data quality metrics reports to the data governance team. These reports help governance teams assess whether data is being managed in accordance with established policies. Data management also plays a role in identifying data issues and suggesting improvements to governance policies.
Collaboration and communication
Effective interaction between data governance and data management requires collaboration and communication. Data management teams need to communicate any challenges, data quality issues, or compliance concerns to the data governance team, who can then make necessary adjustments to governance policies.
Continuous improvement
Data governance and data management work together in a cycle of continuous improvement. Data management teams can provide feedback on the practicality and effectiveness of governance policies, which can lead to adjustments and refinements in data governance practices.
Risk management
Data governance helps identify and mitigate data-related risks, while data management implements controls and measures to reduce these risks. Together, they work to ensure that data availability is used and managed to minimize the potential for data breaches, data loss, and compliance violations.
Conclusion
Data governance and management goals are the same, even though they are two different things. It is essential to have data governance and management in place to ensure that your organizational data can be effectively and beneficially utilized its data in the long run.
QuestionPro is much more than just survey software; we have a solution for every problem and industry. We also have platforms for managing data, such as our InsightsHub research library.
Organizations worldwide are using knowledge management systems and tools like InsightsHub to manage data better, reduce the time it takes to get insights and improve the use of historical data while lowering costs and increasing ROI.