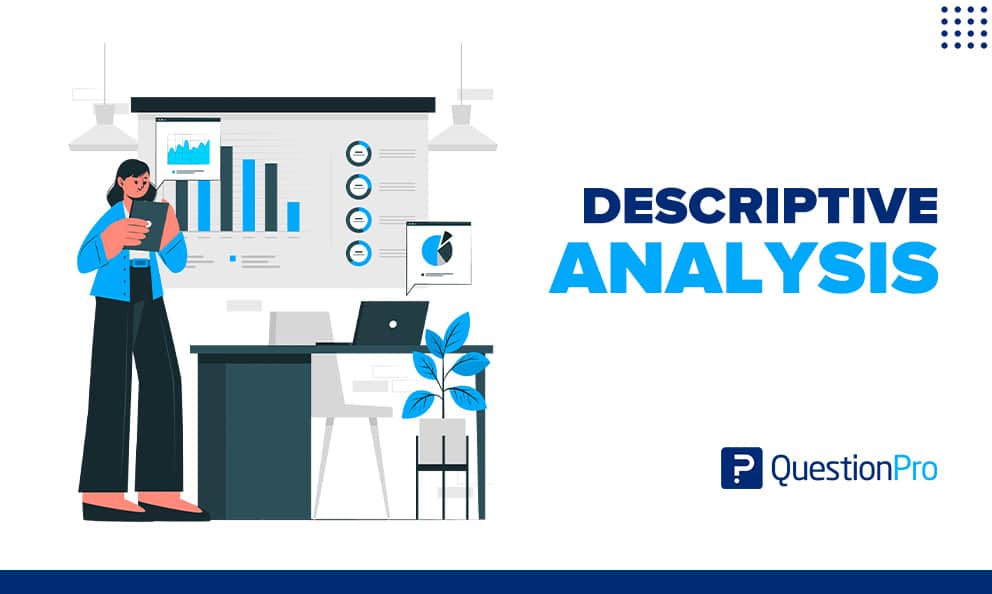
Leading statistical analysis usually begins with a descriptive analysis. It is also known as descriptive analytics or descriptive statistics. It helps you think about how to utilize your data, help you identify exceptions and mistakes, and see how variables are related, putting you in a position to lead future statistical research.
Keeping raw data in a format that makes it easy to understand and analyze, i.e., rearranging, sorting, and changing data so that it can tell you something useful about the data it contains.
Descriptive analysis is one of the most crucial phases of statistical data analysis. It provides you with a conclusion about the distribution of your data and aids in detecting errors and outliers. It lets you spot patterns between variables, preparing you for future statistical analysis.
In this blog, we will discuss descriptive analysis and the best tips for researchers.
What is Descriptive Analysis?
Descriptive analysis is a sort of data research that aids in describing, demonstrating, or helpfully summarizing data points so those patterns may develop that satisfy all of the conditions of the data.
It is the technique of identifying patterns and links by utilizing recent and historical data. Because it identifies patterns and associations without going any further, it is frequently referred to as the most basic data analysis.
When describing change over time, this analysis is beneficial. It utilizes patterns as a jumping-off point for further research to inform decision-making. When done systematically, they are not tricky or tiresome.
Data aggregation and mining are two methods used in descriptive analysis to generate historical data. Information is gathered and sorted in data aggregation to simplify large datasets. Data mining is the next analytical stage, which entails searching the data for patterns and significance. Data analytics and data analysis are closely related processes that involve extracting insights from data to make informed decisions.
Types of Descriptive Analysis
A variety of empirical methodologies support practical descriptive analyses. The most popular descriptive work tools are simple statistics representing core trends and variations (such as means, medians, and modes), which may be highly useful for explaining data.
It is the responsibility of the descriptive researcher to condense the body of data into a form that the audience will find helpful. This data reduction does not mean a situation or phenomenon should be equally weighted in all its components.
Instead, it concentrates on the most critical aspects of the phenomenon as it is and, more generally, the context of real-world practice in which a research study is to be read. The four types of descriptive analysis methods are:
01. Measurements of Frequency
Understanding how often a particular event or reaction is likely to occur is crucial for descriptive analysis. The main goal of frequency measurements is to provide something like a count or a percentage.
02. Measures of Central Tendency
Finding the central (or average) tendency or response is crucial in descriptive analysis. Three standards—mean, median, and mode—are used to calculate central tendency.
03. Measures of Dispersion
At times, understanding how data is distributed throughout a range is crucial. This kind of distribution may be measured using dispersion metrics like range or standard deviation.
04. Measures of Position
Finding a value’s or response’s location concerning other matters is another aspect of descriptive analysis. In this area of knowledge, metrics like quartiles and percentiles are beneficial.
How to Conduct a Descriptive Analysis?
Descriptive analysis is an important phase in data exploration that involves summarizing and describing the primary properties of a dataset. It provides vital insights into the data’s frequency distribution, central tendency, dispersion, and identifying position. It assists researchers and analysts in better understanding their data.
Conducting a descriptive analysis entails several critical phases, which we will discuss below.
Step 1: Data Collection
Before conducting any analysis, you must first collect relevant data. This process involves identifying data sources, selecting appropriate data-collecting methods, and verifying that the data acquired accurately represents the population or topic of interest.
You can collect data through surveys, experiments, observations, existing databases, or other methods.
Step 2: Data Preparation
Data preparation is crucial for ensuring the dataset is clean, consistent, and ready for analysis. This step covers the following tasks:
- Data Cleaning: Handle missing values, exceptions, and errors in the dataset. Input missing values or develop appropriate statistical techniques for dealing with them.
- Data Transformation: Convert data into an appropriate format. Examples of this are changing data types, encoding categorical variables, or scaling numerical variables.
- Data Reduction: For large datasets, try reducing their size by sampling or aggregation to make the analysis more manageable.
Step 3: Apply Methods
In this step, you will analyze and describe the data using a variety of methodologies and procedures. The following are some common descriptive analysis methods:
- Frequency Distribution Analysis: Create frequency tables or bar charts to show the number or proportion of occurrences for each category for categorical variables.
- Measures of Central Tendency: Calculate numerical variables’ mean, median, and mode to determine the center or usual value.
- Measures of Dispersion: Calculate the range, variance, and standard deviation to examine the dispersion or variability of the data.
- Measures of Position: Identify the position of a single value or its response to others.
Identify which variables are important to your descriptive analysis and research questions. Various methods are used for numerical and categorical variables, so it is essential to distinguish between them.
After the data set has been analyzed, researchers may interpret the findings in light of the goals. The analysis was successful if the conclusions were what was anticipated. Otherwise, they must search for weaknesses in their strategy and repeat these processes to get better outcomes.
Step 4: Summary Statistics and Visualization
Descriptive statistics refers to a set of methods for summarizing and describing the main characteristics of a dataset. Summarize the data through statistics and visualization. This step involves the following tasks:
- Summary Statistics: Summarize your findings clearly and concisely.
- Data Visualization: Use various charts and plots to visualize the data. Create histograms, box plots, scatter plots, or line charts for numerical data. Use bar charts, pie charts, or stacked bar charts for categorical data.
Best Research Tips to Complete Descriptive Analysis
Moreover, what researchers can do to complete descriptive analysis are:
- They must specify the purpose of the in-depth analysis, the goals, the direction they will take, the things they must overlook, and the format in which the data must be provided.
- They must gather data after identifying the goals. This is a critical phase since collecting incorrect data might lead them far from their objective.
- Cleaning up the data is the next stage. When working with massive data sets, data cleansing may become challenging. The working data set’s noise or irrelevant information might skew the findings. Researchers should clean the data following the specifications for reliable results.
- Different descriptive techniques are used once the data has been cleaned. In the form of in-depth descriptive summaries, the descriptive analysis highlights the fundamental characteristics of the data.
- After the data set has been analyzed, researchers may interpret the findings in light of the goals. The analysis was successful if the conclusions were what was anticipated. Otherwise, they must search for weaknesses in their strategy and repeat these processes to get better outcomes.
- When you’re presenting your analysis to non-technical stakeholders and teams, it might be challenging to communicate the findings. Data visualization helps to complete this task efficiently. To give the results, researchers might use a variety of data visualization approaches, such as charts, pie charts, graphs, and others.
Conclusion
Descriptive analysis is a crucial research approach, regardless of whether the researcher wants to discover causal relationships between variables, explain population patterns, or develop new metrics for basic phenomena. When used correctly, it may significantly contribute to various descriptive and causal research investigations.
Looking at the correct data and evaluating it is pretty valuable for researchers and marketers. You may gather research data and execute complex analysis within the tool with an established research platform like QuestionPro, which enables you to get the insights that matter.
Using QuestionPro, you can quickly reach important decisions while better understanding your customers and other research objects. Utilize the enterprise-grade research suite’s capabilities right now!