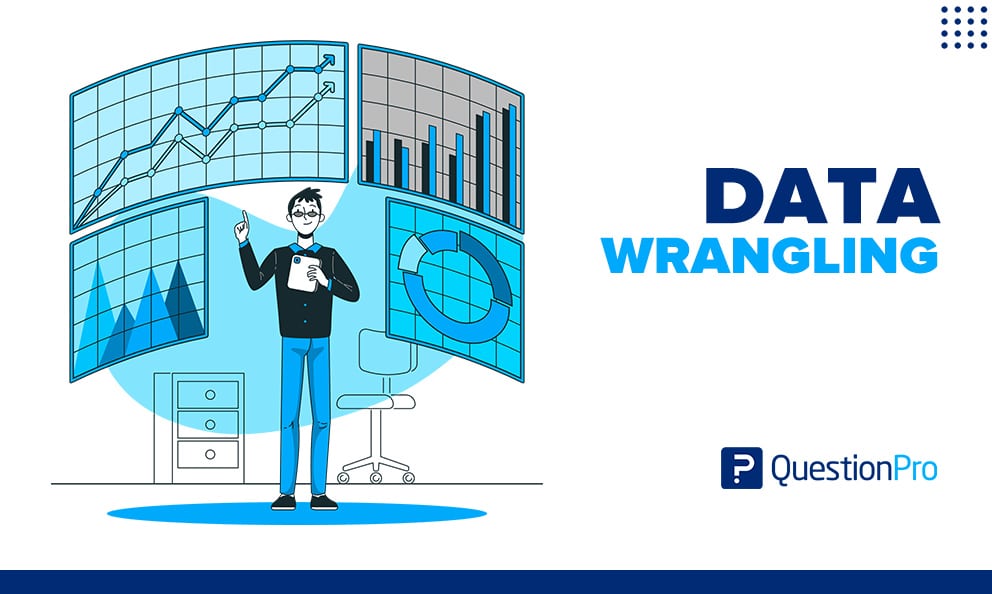
In today’s digital age, businesses obtain vast volumes of data online. Raw data should be processed efficiently and carefully. Here comes data wrangling, and it is used to assist in the transformation of raw data into valuable data that can provide informative results.
You can make better business judgments with the help of data wrangling if you do it correctly. You may learn about data wrangling here, the steps involved, and the best practices that go along with it. So, let’s get this started!
What is data wrangling?
Data wrangling is the process of transforming raw data into a more processed shape by reorganizing, cleansing, and enriching it. Data wrangling entails processing data in various formats and analyses and combining them with another data set to produce meaningful insights. The specific strategies vary based on the data you’re utilizing and the aim you’re attempting to achieve.
The following are examples of data wrangling:
- Combining data sources for analysis.
- Filling or removing data gaps.
- Deleting unnecessary or irrelevant project data.
- Identifying data outliers and explaining or deleting them to allow analysis.
Data wrangling can be done manually or automatically. When datasets are enormous, it’s essential to clean them automatically. A data scientist or other dedicated team member is often in charge of data wrangling in businesses with a comprehensive data team. Smaller companies frequently rely on non-data specialists to clean their data before using it.
What are the benefits of data wrangling?
Wrangling the data is beneficial. When you consider how beneficial it will be, it is clear that it is worth your time to put in the effort to understand it. The following are some benefits that data wrangling can provide for your business:
- Simple analysis: Business analysts and stakeholders may examine even the most complex data quickly, efficiently, and effectively once raw data has been tamed and converted.
- Data handling: The procedure turns raw, unstructured data into rows and columns. The technique enriches the data to gain a deeper understanding.
- Improved targeting: Combining data from several sources helps you better understand your audience, which improves the targeting of your ad campaigns and content strategy.
- Use of time: The technique allows analysts to spend less time managing disordered data and more time acquiring insights to make accurate decisions based on simple-to-understand data.
- Data visualization: The data may be exported to any visual analytics platform to sort, analyze, and summarize the data once it has been wrangled.
Necessary steps to perform data wrangling
Each data project needs a different strategy to guarantee that the final dataset is trustworthy and available. These are frequently referred to as necessary data wrangling stages or activities.
Step 1: Discovery
The discovery process is the initial step in the data wrangling process. It is a step toward gaining a better understanding of the data. To make your data easier to use and analyze, you must look at it and consider how you would like the data to be arranged.
The data may show trends or patterns during the discovery process. This is a crucial step because it will influence all subsequent actions. It also identifies obvious problems, like values that are missing or incomplete.
Step 2: Structuring
Most of the time, incomplete or improperly formatted raw data is unsuitable for the intended purpose. The process of taking unprocessed data and converting it so that it may be used more easily is known as data structuring.
This is the method for extracting relevant information from new data. The data can be structured in a spreadsheet by adding columns, classes, headings, etc. This will improve the usability so the analyst can easily use it in his analysis.
Step 3: Cleaning
Cleaning up data involves eradicating any ingrained flaws that could skew your analysis or reduce its usefulness. Data cleaning or remediation aims to ensure that the final data for analysis is not impacted.
Raw data usually contains errors that must be cleaned before it can be used. Data cleaning includes correcting outliers, deleting bad data, etc. When cleaning the data, you get the following results:
- It removes outliers that can bias data analysis results.
- It changes data type and simplifies data to increase quality and consistency.
- It finds duplicate values, eliminates structural problems, and verifies data to make it easier to use.
Step 4: Enriching
Adding context to the data is what is meant by enriching. This process transforms previously cleaned and formatted data into new types. At this point, you need to plan strategically for the information you already have to get the most out of it.
Downsampling, upsampling, and then auguring the data is the best way to get it in its most refined form. If you feel that enrichment is necessary, you will need to repeat the methods for any additional data you obtain. The step of enriching the data is optional. If the data you already have doesn’t meet your needs, you can go through this step.
Step 5: Validating
Repeated programming steps are required to ensure that the data is correct, consistent, safe, and authentic. The process of ensuring that your data is accurate and consistent is known as data validation. This step can reveal problems that need to be fixed or conclude that the data is ready for analysis.
Step 6: Publishing
Publishing is the last step in data wrangling, showing what the whole process is all about. It’s about putting the new wrangled data in a place where you and other stakeholders can easily find and use it. The information can be added to a fresh database. As long as you follow the previous steps, you’ll have high-quality data for insights, business reports, and more. Practical business intelligence relies on the synergy between analytics and reporting, where analytics uncovers valuable insights, and reporting communicates these findings to stakeholders.
Data wrangling best practices
It is possible to execute data wrangling in a variety of methods. The methods can differ depending on the targeted audience for which the data is being presented. The following is a list of some recommended practices that are applicable in every circumstance:
Get a better understanding of your audience
The unique needs of data wrangling are company-specific. It is crucial to identify who will access and analyze the data and what they intend to achieve. This way, you can get useful information about your audience to learn more about them.
For example, you can get all the demographic information about your current customers so that the marketing team knows who to target with their advertising.
Select the appropriate data
It’s not about having a lot of data; it’s about having the correct data. That is why data selection is so critical. Here are some pointers for selecting the appropriate data:
- Avoid using data that contains a large number of nulls or numbers that are the same or repeated.
- Stay away from values that have been calculated and choose data that are closer to the source.
- Gather information from a number of different types of platforms.
- Apply certain filters to the data, and then choose a topic that satisfies the requirements and guidelines.
Understand the data
You need to understand how the data complies with your organization’s governance principles and guidelines. Observe the following significant facts:
- Gain an understanding of the data, database, and file types.
- Explore the present condition of the data by using the features provided by visualization tools.
- Create data quality metrics by using characterization.
- Be careful of the limits of the data.
Adopt newly developed tools and techniques
Every day, new technologies are being combined with existing ones, and audiences continue to expand. Data experts must adapt to new tools and analytics technology to provide efficient data wrangling services.
Conclusion
Data wrangling has become increasingly important in recent years due to the massive amounts of data that are handled daily to improve user experiences. The business would suffer without a strong data storage system and investments in data wrangling techniques. You should now have a better understanding of data wrangling and the processes involved due to this article.
At QuestionPro, we provide all the tools required for researchers to complete their tasks successfully. It will walk you through the process to get the most value out of your data.