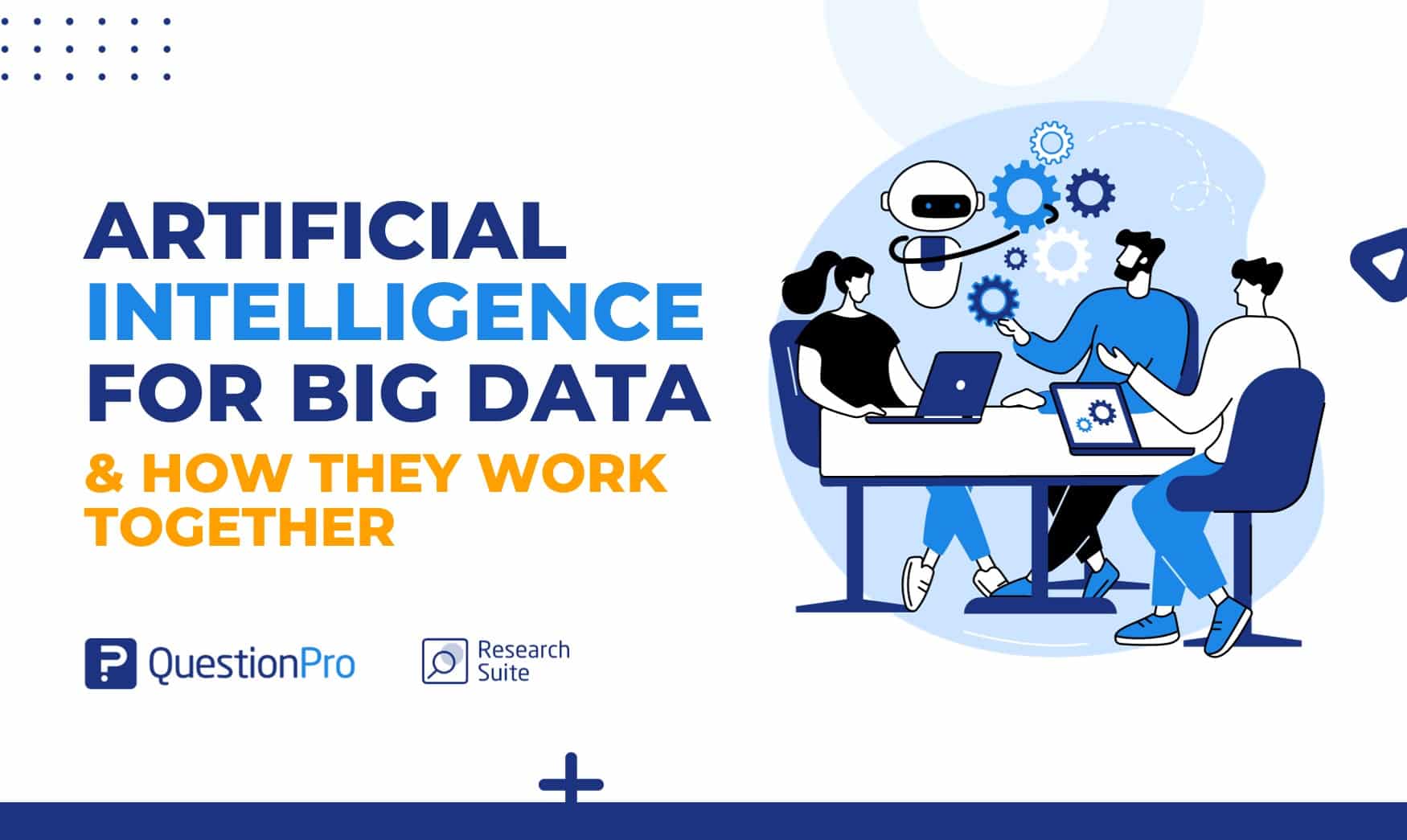
Most businesses are constantly inundated with massive amounts of information. This influx of data presents both opportunities and challenges. On one hand, it holds the potential to provide valuable insights that can drive strategic decisions and innovation. On the other hand, the sheer volume and complexity of this data can be overwhelming. This is where Artificial Intelligence for Big Data connects, offering a powerful solution to get meaningful insights from data.
What is Artificial Intelligence for Big Data?
Artificial Intelligence for Big Data, often referred to as AI in Big Data or AI for Data Analytics, is the fusion of two cutting-edge technologies: Artificial Intelligence and Big Data. It involves using AI-driven algorithms and machine learning techniques to analyze, interpret, and derive actionable insights from large and complex datasets. The primary goal of AI in Big Data is to automate and enhance the process of data analysis, making it faster, more accurate, and scalable.
At its core, AI for Big Data leverages machine learning models that can recognize patterns, make predictions and continuously improve their performance with minimal human intervention. These models are trained on datasets, allowing them to identify trends, anomalies, and correlations that might be impossible or extremely time-consuming for humans to uncover. By doing so, AI for Big Data empowers organizations to turn raw data into strategic assets, driving informed decision-making and gaining a competitive edge in their respective industries.
How Big Data and AI Work Together
Big Data and AI are not just complementary; they are interdependent. Big Data provides the raw material, the vast datasets, for AI to work its magic. The synergy between the two can be illustrated in the following steps:
- Data Collection: Big Data encompasses the collection of vast amounts of structured and unstructured data from various sources, including sensors, social media, customer interactions, and more. This data forms the foundation for AI applications.
- Data Storage and Processing: Big Data technologies, such as Hadoop and Spark, facilitate the storage and processing of massive datasets. This infrastructure ensures that the data is accessible and available for AI algorithms.
- Data Preprocessing: Before AI can analyze the data, it often requires preprocessing. This step involves cleaning, transforming, and structuring the data to make it suitable for machine learning models.
- AI Modeling: Machine learning algorithms, a subset of AI, are then applied to the prepared data. These algorithms can include supervised learning for prediction, unsupervised learning for pattern recognition, and reinforcement learning for decision-making.
- Training and Inference: AI models are trained on historical data to learn patterns and relationships. Once trained, they can make predictions or decisions based on new, incoming data in real time.
- Insight Generation: The final output of this process is actionable insights. AI algorithms reveal hidden patterns, anomalies, trends, and predictions from Big Data, which can be used for various purposes, from improving products and services to optimizing business operations.
What is the Best AI for Big Data?
When it comes to choosing the right AI for Big Data, there is no one-size-fits-all solution. The selection depends on the specific needs and objectives of an organization. However, several AI technologies have gained prominence in the realm of Big Data analytics:
- Machine Learning: Machine learning is a fundamental component of AI for Big Data. It includes various techniques like supervised learning, unsupervised learning, and deep learning. Supervised learning, for instance, is used for classification and regression tasks, making it suitable for predictive analytics with Big Data.
- Natural Language Processing (NLP): NLP is a subset of AI that focuses on the interaction between computers and human language. It’s particularly valuable for analyzing unstructured textual data, such as customer reviews, social media posts, or news articles, at scale.
- Computer Vision: Computer vision enables machines to interpret and understand visual information from the world, including images and videos. This technology is invaluable for tasks like image recognition, object detection, and facial recognition, which can be applied to Big Data scenarios.
- Reinforcement Learning: In cases where decision-making is crucial, reinforcement learning algorithms can be employed. They are well-suited for optimizing complex systems and processes, such as supply chain management or autonomous vehicles, by learning through interaction.
- Deep Learning: Deep learning, a subset of machine learning, involves neural networks with multiple layers. It’s especially effective for tasks that require high accuracy in pattern recognition, such as speech recognition or image classification.
Selecting the best AI technology depends on the specific goals of your Big Data analytics project. In many cases, a combination of these AI techniques may be required to extract the most valuable insights from diverse datasets.
Examples of Artificial Intelligence for Big Data
Artificial Intelligence (AI) plays a central role in Big Data, contributing in several essential ways. AI-driven algorithms automate the data analysis process, resulting in significant time savings and reduced human error. These algorithms efficiently handle vast datasets, unveiling hidden patterns and trends that might otherwise remain unnoticed.
It also excels in predictive analytics, utilizing historical data to make informed predictions. Whether forecasting customer behavior, equipment failures, or market trends, AI empowers decision-making with actionable insights. They are adept at detecting anomalies within datasets, a critical capability for tasks like fraud detection, network security, and quality control.
AI-powered recommendation systems leverage Big Data to offer personalized content and product suggestions, as exemplified by Netflix and Amazon. Lastly, Natural Language Processing (NLP) in AI enables organizations to analyze and comprehend customer sentiment, feedback, and textual opinions, contributing to product and service enhancements.
AI for Big Data has made significant impacts across various industries:
- Healthcare: AI is used to analyze patient data, assist in diagnosing diseases, predict patient outcomes, and even personalize treatment plans based on individual health records.
- Finance: Financial institutions utilize AI for fraud detection, algorithmic trading, credit risk assessment, and customer service chatbots.
- Retail: AI-driven recommendation engines personalize shopping experiences, optimize inventory management, and provide dynamic pricing strategies.
- Manufacturing: Predictive maintenance powered by AI reduces downtime by forecasting equipment failures, while quality control systems enhance product quality.
- Marketing: AI enhances marketing campaigns by analyzing customer behavior, segmenting audiences, and optimizing ad targeting.
Artificial Intelligence for Big Data: Similarities and Differences
Artificial Intelligence for Big Data is a formidable combination that empowers organizations to extract value from their vast and complex datasets. By harnessing the capabilities of AI-driven algorithms, businesses can automate data analysis, gain predictive insights, and uncover hidden patterns that drive informed decision-making.
While AI and Big Data are distinct fields, they share commonalities and differences:
Similarities:
- Data-Driven: Both AI and Big Data rely on data as their lifeblood. AI requires large datasets for training, and Big Data is the source of these datasets.
- Machine Learning: AI heavily employs machine learning, which is a subset of both fields. Machine learning models are trained on Big Data to make predictions and decisions.
Differences:
- Scope: Big Data focuses on collecting, storing, and processing large volumes of data, while AI is concerned with creating algorithms and models for tasks like pattern recognition and decision-making.
- Purpose: Big Data’s primary purpose is to manage and analyze data, while AI’s purpose extends to creating intelligent systems that can perform tasks autonomously.
In essence, Big Data provides the raw material, and AI processes and interprets that material to generate insights and drive intelligent actions.
Conclusion
The ability to turn data into a strategic asset is a game-changer. It allows organizations to enhance customer experiences, optimize operations, and stay ahead of market trends. As AI continues to advance and Big Data continues to grow, the synergy between the two will unlock new possibilities, enabling businesses to thrive in the era of data-driven intelligence.
Embracing this synergy can lead to a future where organizations not only survive but thrive in a data-rich world. So, the question is not whether to adopt AI for Big Data, but how soon and effectively to embark on this transformative journey.
Explore the cutting-edge capabilities of QuestionPro’s QxBot and unlock the full potential of AI-powered analytics for your big data needs.
QxBot is QuestionPro’s innovative generative AI tool designed within our existing survey platform. Using a conversational interface to create surveys on any topic within seconds, you can build quick, creative surveys on any topic you are looking to research in as little as 60 seconds.
Discover how QxBot can streamline data analysis, enhance decision-making, and provide actionable insights. Dive into the future of AI by giving QxBot a try today!