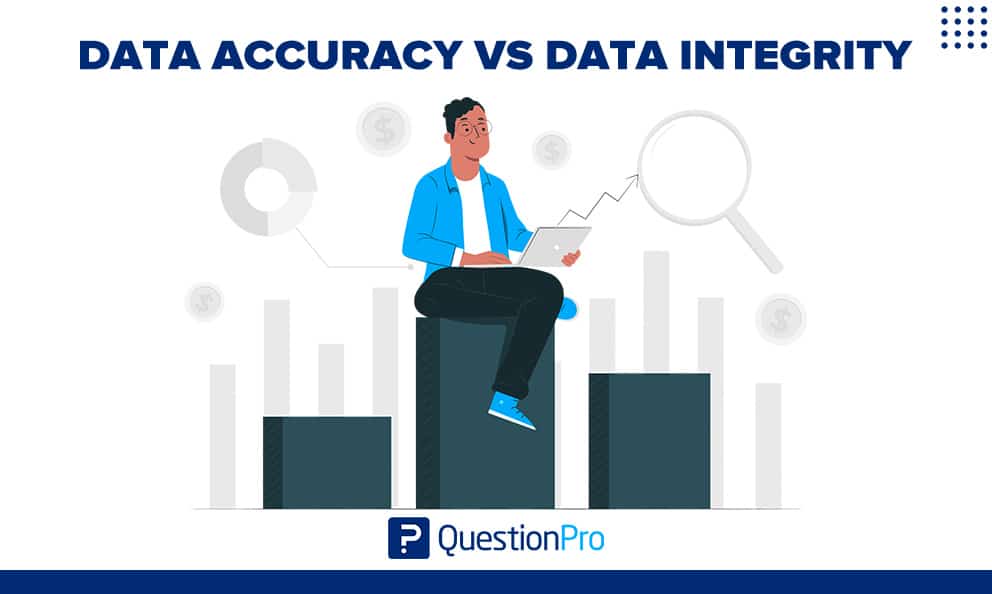
Data may be your organization’s data most valuable asset. If your organization relies on gathering, storing, and using data, then keeping that data safe, secure, and correct is critical to your business’s capacity to survive and develop. Unreliable data can lead to wrong conclusions, distorted analyses, and imprudent recommendations. Let’s discuss data accuracy vs data integrity.
People frequently mix up data integrity with accuracy, even though they are distinct. Businesses need to keep data integrity high, but tracking everything and ensuring data is correct across different departments and data sets can be challenging.
This blog post will discuss data accuracy vs. data integrity and how they differ.
Data Accuracy
The most crucial part of data quality is accuracy. It ensures that your company’s business decisions are based on reliable and accurate data. This makes making good decisions in all areas easier, like planning, predicting, budgeting, etc. The internal validity of the information is referred to as accuracy. It suggests that the data is accurate and error-free.
Completeness is a component of accuracy as well. If you only have partial information, it’s possible that you won’t be able to get to the correct conclusions regarding an issue or a problem.
Let’s imagine you wish to keep tabs on inventory levels as a retailer. If you have complete data collection, you can ensure that you always have adequate inventory to satisfy customer demand. You may also use data accuracy to predict trends to acquire stock ahead of time and keep one step ahead of the competition.
Data integrity
Data integrity refers to how well data is stored throughout its life cycle. It relates to the accuracy with which data is entered into a system. This signifies that the information is complete, consistent, and correct.
Data analytics and data analysis are closely related processes that involve extracting insights from data to make informed decisions. It involves more complex techniques like predictive modeling, machine learning, and data mining to provide actionable insights for strategic planning and decision-making.
You should consider how you will handle data integrity when creating a database. For instance, if you want to store customer information in a relational database, you must ensure that two customers do not have the same name. To do this, you could give each customer a unique number.
Data integrity protects against data loss and leaks. To protect your data from hostile outsiders, make sure internal users handle it properly. Data validation and error checking may ensure that sensitive data isn’t miscategorized or stored wrongly.
Data integrity has several metrics to consider:
- Data accuracy: How accurate the data is. 95% data correctness indicates the data closely matches the actual set.
- Data completeness: How much data is stored within a data set.
- Data security: Keeping data secure against illegal access.
- Data governance: Ensuring data meets organization needs.
- Data validity: Checking for faults to ensure that data is valid.
- Data uniqueness: Ensure that the data is unique.
- Location intelligence: Location insight and analytics enrich and make data actionable.
- Data enrichment: External data adds context, nuance, and relevance to internal data. Including business, consumer, or location details improves your data’s completeness and context.
LEARN ABOUT: Data Mining Techniques
Importance of Data accuracy and Data integrity
Any company needs data accuracy and integrity to help ensure that the data is comprehensive, consistent, and accurate. Data accuracy is important for a business because it provides correct and up-to-date information. This makes it easier to make business decisions and plan strategically.
Data integrity is essential because it makes sure the information hasn’t been changed and is still correct. This helps keep the trust of customers and clients and protects the company’s reputation. It also helps to confirm the legitimacy of the data, which is an essential step in preventing the data from becoming corrupted.
LEARN ABOUT: Data Asset Management
Data Accuracy vs Data Integrity Differences
Data accuracy and integrity are two critical aspects of data management. Let’s take a look at how they differ from one another.
Data Accuracy | Data Integrity |
Data accuracy indicates the data’s overall quality. | Data integrity is defined as having accurate and comprehensive data. |
It’s important for businesses to make smart decisions. | It is important to make sure that the information has not been changed or lost. |
It is necessary to have procedures in place for entering, managing, and safeguarding information to preserve data accuracy. | To ensure data integrity, additional governance and security procedures may be required. |
It might be challenging to ensure the accuracy of data across multiple departments and data types. | Data integrity is simply maintaining the accuracy and completeness of a data set. |
Accuracy, completeness, consistency, and error rates are crucial data accuracy metrics. | Data integrity metrics include data quality, completion, safety, real time governance, correctness, originality, location intelligence, and enrichment. |
The risks of inaccurate data include incorrect decision-making, reputational damage, and failure to comply. | Not having data integrity risks include financial losses, wrong diagnosis, and overpaying for insurance. |
LEARN ABOUT: Data Management Software
Conclusion
We know that data is critical, and data accuracy vs data integrity are two crucial parts of data storage. Accuracy refers to how accurate the data is, whereas integrity refers to whether or not the data has been changed.
The problem is that many companies put accuracy ahead of integrity, which can lead to severe consequences. The importance of data accuracy and integrity cannot be overstated because they help to ensure that data is accurate.
QuestionPro is more than just survey software because it provides solutions for various issues and industries. For example, our InsightsHub research library is a platform for storing and analyzing data.
Companies worldwide are turning to systems like InsightsHub’s knowledge management tools and platforms to improve data management, speed up insight generation, and better use of historical data, all while reducing costs and increasing return on investment.