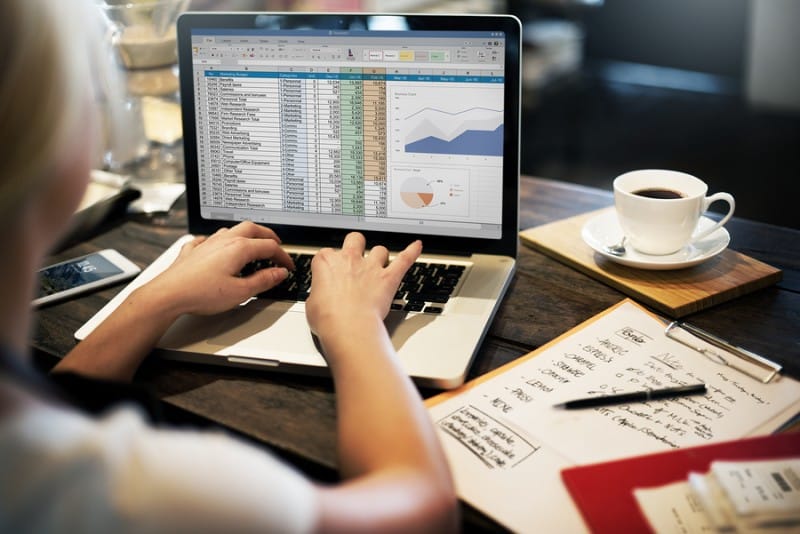
In recent posts, we examined the nature of the data types available to consumer or B2B market researchers including; nominal, ordinal, interval and ratio. The latter two categories allow the user to generate mean score or averages as part of their survey data analysis. Working with means gives the researcher access to a wealth of multivariate statistics, but mean scores are not without their issues. Let’s look at a few of them.
First, means are heavily influenced by extreme scores, also known as outliers. These high and/or low scores can pull the mean up or down depending upon their location. This is why variables that focus on wealth, income or prices are typically reported using the median, e.g. the median housing price or median monthly income. Variables with numerical categories such as customer satisfaction or brand awareness tend to be less influenced by outliers. Options for dealing with outliers include removing them from analysis or recoding them with the median value. Either option is not without considerations that need to be addressed. These are beyond the scope of this post.
Another concern is the overall distribution of the datapoints. If half of the survey respondents say they like their coffee hot while the other half say they like it cold, then the ‘mean’ is going to be in the middle or lukewarm. In cases such as this, the mean or average would be misleading. Further commentary by the researcher outlining the distribution of the data is called for. This highlights the distribution and makes it easier for the decision-maker to derive understanding.
In survey research, be it market or otherwise, if we report the mean score then we should also report the standard deviation, the median, and possibly the range (high – low) as well. These supporting statistics provide the end-user a picture to better understand the nature of the data. A graphic can also be useful.
Within all consumer data lays variation. This variability can show up clearly when looking at mean scores if you have the right filter applied.
Learn more about working with mean scores by reviewing Data Analysis.