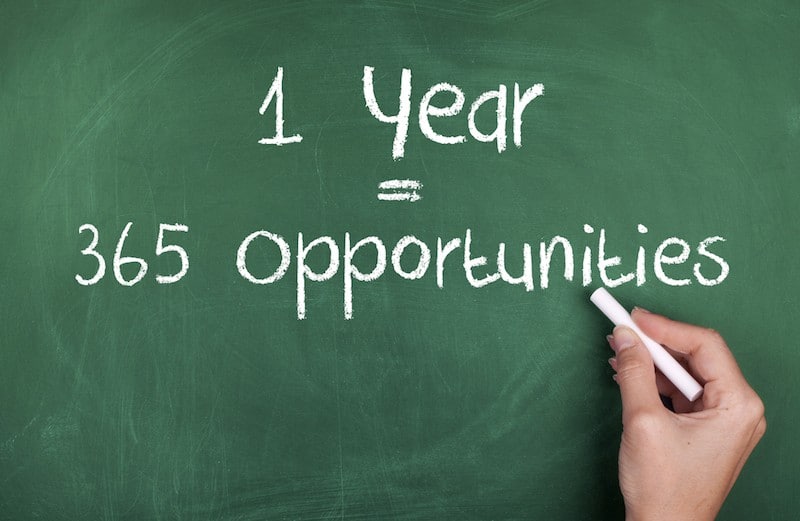
When considering the type of data needed for a survey question we have several choices; nominal data, ordinal data, interval data and ratio data. In addition to the type of data, most survey platforms offer the survey author a wide variety of visual presentations. This post focuses on the use of numerical scales versus ordinal for measuring optimism and other useful constructs.
As market researchers, we are often interested in a respondent’s perceived level of optimism. Is the optimistic respondent that is more likely to purchase our products and services, either now or at some specified point in the future? Does the question then become how best to measure optimism?
Using an ordinal scale we could provide the respondent with a question like this:
How optimistic are you about the current business conditions facing your company?
Not optimistic at all
Somewhat optimistic
Optimistic
Very optimistic
This scale produces an ordinal measure of the respondent’s view of the conditions their company is facing. Collectively, we can use percentages to measure the overall sense of optimism. Optimistic groups could be separated from non-optimistic groups by incorporating other survey questions (business size, industry, geographic region etc.). On the plus side, this type of scale is easy on the respondent, which can lead to data that is more reliable. On the downside, due to its ordinal nature, our hand is forced when looking at analytical options.
Alternatively, we could ask a respondent to rate their level of optimism on a scale from 0 to 100, with 0 being not optimistic at all and 100 being very optimistic. Having an absolute zero point produces ratio data, from which the full power of statistics can be applied. This is the upside. We can now conduct a regression analysis or use other multivariate techniques to isolate the key drivers to optimism.
The main detractor is an increased cognitive load. With a scale this wide (0 – 100), respondents may not make use of the full range of the scale leading to clusters of data points and a non-normal distribution, which can be a limiting factor with multivariate statistics. One way to mitigate this is to offer a visual slider with endpoints clearly marked. Invariably the survey author will also need to make mental cutoff points to facilitate data analysis.
As in all forms of research, there are tradeoffs to be made which will impact data quality and facilitate comparison of your survey data to other published measures. Depending upon your audience and analytical needs the ordinal scale may be sufficient. In other cases, the robustness of a ratio measure may outweigh the higher level of cognition needed to answer accurately. The key takeaway is there are options for measuring optimism, satisfaction, awareness and other constructs of interest to marketers.
For more insight into working with metric data see – Data Analysis 101 – Metric Data
For a primer on using surveys see – Survey Data Analysis