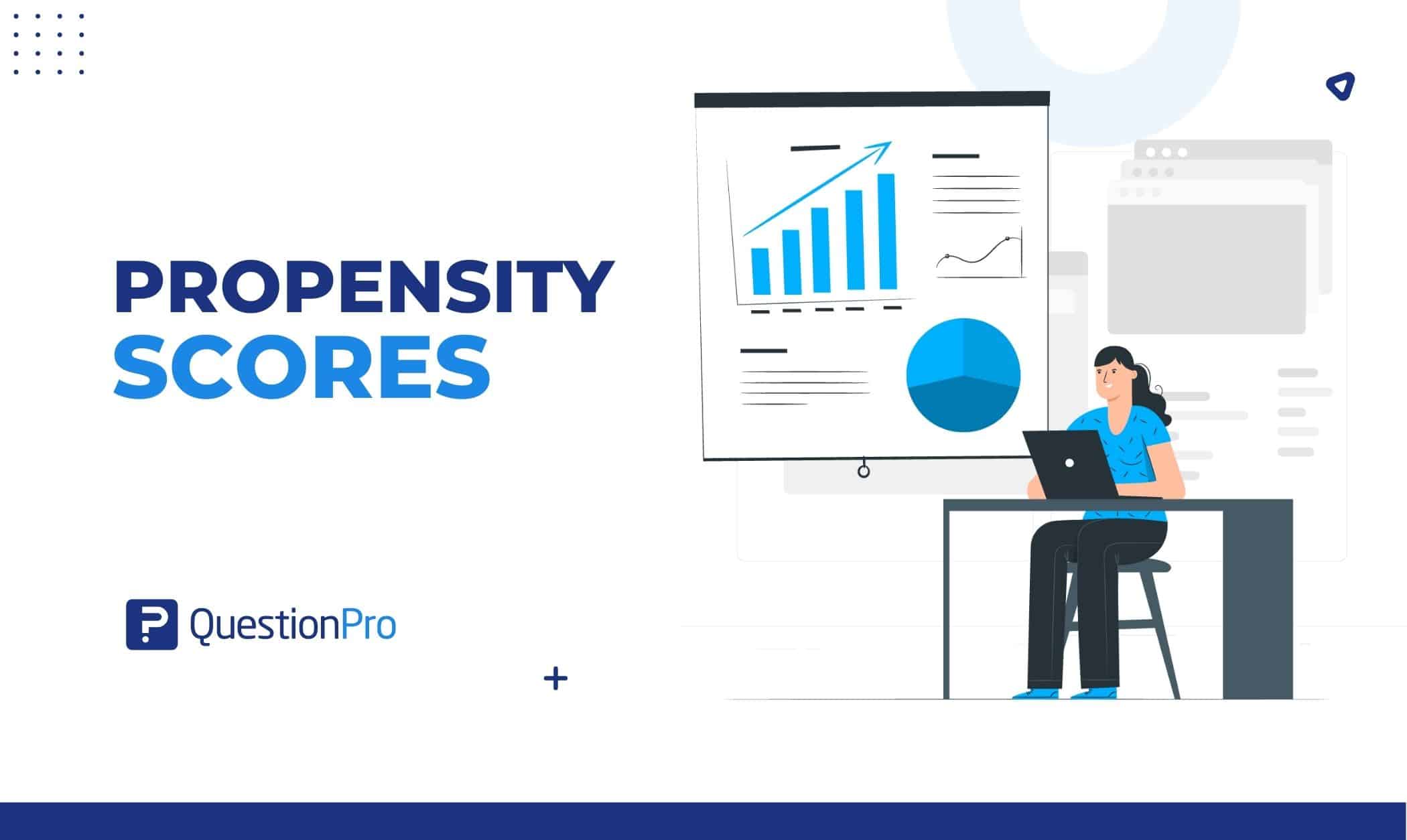
A propensity score is helpful when using observational data to estimate the effect of a treatment on an outcome and when it is likely that there will be selection bias because the treatment wasn’t given at random. It is increasingly employed in cardiovascular research and has been used to eliminate bias in observational studies in various domains.
Since its initial publication in 1983, these scores have become widely used to support causality in research that cannot employ random assignment. The concepts underlie these methodologies’ utilization are complex, even though their utility is significant.
And in this blog, we will explain the techniques of construction and evaluation of propensity score.
What are propensity scores?
The propensity score is the likelihood that a treatment will be assigned based on observed baseline characteristics. It is a balancing score since it determines whether the distribution of recorded baseline covariates is equal between treated and untreated participants. A nonrandomized observational study can be designed and analyzed using the propensity score to resemble some of the unique features of a randomized controlled trial.
When the random assignment to a condition is impractical, scores give an alternative to account for confounding. Because of imbalances between the treatment and control groups when the random assignment cannot be used, the effect of the treatment condition (also known as the grouping variable) on the outcome is biased.
Propensity scores are commonly utilized in cardiovascular research and have been used to eliminate bias in observational studies in various domains.
Techniques of construction and evaluation of propensity scores
- Choice of Variables for the Propensity Score
Propensity ratings include treatment and outcome variables to reduce confounding. The first step in creating a propensity score is to employ a logit or probit regression analysis with treatment as the outcome variable and potential confounders as explanatory variables.
Covariate selection balances bias and efficiency. The propensity score should reduce bias if a variable is related to the outcome but not the treatment because a treatment-related variable may affect the outcome.
Caution: Exclude treatment-related variables. A treatment-affected propensity score hides part of the treatment impact. Exclude covariates that perfectly predict treatment status because treatment and comparison group covariate distributions must overlap.
- Propensity score balance between the comparison and treatment groups
After calculating each observation’s score, one must guarantee that the treatment and comparison groups’ scores overlap.
Treatment effects cannot be inferred for a treated individual without a comparable propensity score. Standard support is subjectively judged using a graph of propensity scores across treatment and comparison groups.
Caution: Propensity scores balance measured factors, not unmeasured ones. Unmeasured variables can skew treatment effect estimates. As measured and unmeasured factors get closer, this bias may rise.
- Balance of Covariates across Treatment and Control Groups within Blocks of the Propensity Score
Propensity score balancing has no ideal technique. After balancing the score across blocks between treatment and comparison groups, verify for covariate balance within blocks. This guarantees that the propensity score is set correctly and distributed similarly across groups within each block.
Balance in the mean does not suggest balance in higher-order moments, but an imbalance in the mean requires respecifying the propensity score. Instead, compute standardized differences.
Caution: Do not use c-statistics or AUC to evaluate performance. These metrics are problematic because they are meant to reduce confounding, not predict.
- Choice of Strategies for Matching and Weighting
After developing a balanced propensity score, choose how to compare treatment and comparison groups. Bias and efficiency tradeoffs must be considered.
The most common comparison procedures are matching and weighting. No matter how weak the match, matching techniques can match a treated individual to the comparison individual with the greatest identical propensity score.
Matching techniques can match a treated person to the comparison person with the most identical propensity score, regardless of the caliper.
- Balance of Covariates Following Matching or Propensity-Score-Based Weighting of the Sample
After establishing a matching or weighting approach, assessing how effectively the treatment and comparison groups are balanced is essential. The propensity score must be prespecified if the treatment and comparison groups are unbalanced.
Comparing standardized differences is a famous first test, like balancing steps. More minor mean differences and higher-order moments are better in confounders anticipated to affect the outcome severely.
Caution: Treatment effect estimates are affected by sample restriction to a common support. A therapy’s effect can only be determined for people with propensity scores in the treatment and comparison groups.
Conclusion
Numerous statistical analyses employ these scores. Given the rising cost of randomized clinical trials and the growing popularity of observational studies as a research methodology, their use is projected to rise over time.
When it can be integrated into the design stages of studies, the methodology tends to yield the most significant benefits (through matching or stratification).
Along with time and money savings, these advantages also include more accurate assessments of therapy effects. By being able to avoid recruiting individuals who might not be suitable for particular investigations, this saving is made possible.
It is an extra tool that researchers have at their disposal when attempting to assess treatments’ effects in studies with a chance of bias. This score is one of many techniques that can be used to analyze data from observational studies.
QuestionPro is more than survey software. Our InsightsHub research library handles data. QuestionPro can help you swiftly make critical judgments by helping you comprehend clients and other issues. Use the enterprise-grade research suite now!