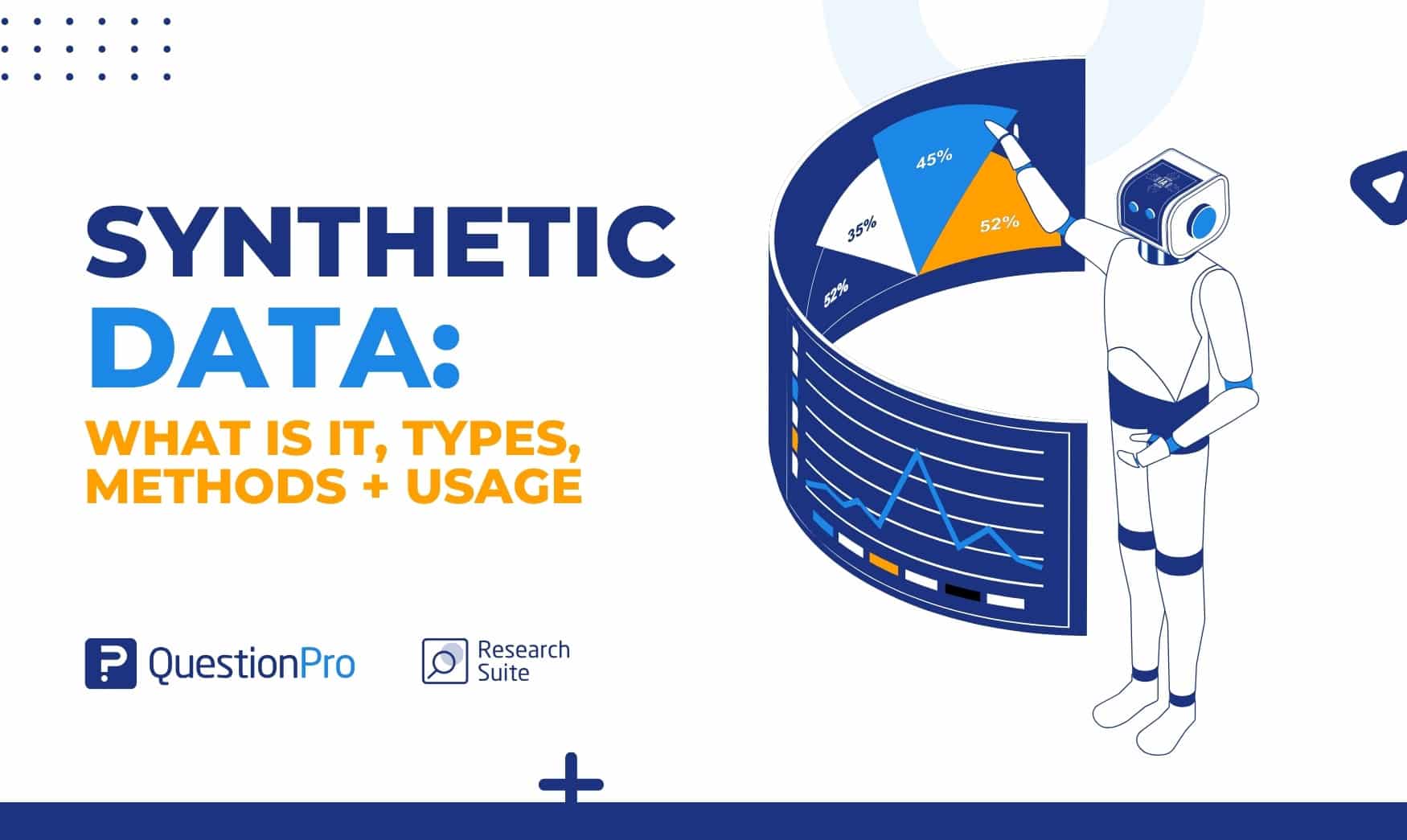
Synthetic data expands the area of research and education. It refers to intentionally manufactured data replicating real-world data’s statistical characteristics in the field of data-driven insights.
You may come upon sensitive datasets that cannot be released openly due to privacy regulations. Synthetic information can help you communicate, build models, and perform tests without exposing personal information.
Stay tuned as we explore the world of synthetic data, uncovering its various types, generating methods, and tools that enable data professionals like you to make informed judgments while respecting privacy and ethical concerns.
What is Synthetic Data?
Synthetic data is artificially generated data that replicates the qualities and statistical properties of real-world data. But it does not contain any actual information from real people or sources. It’s like copying the patterns, trends, and other features found in real data but without any real information.
It is created using various algorithms, models, or simulations to recreate the patterns, distributions, and correlations found in actual data. The goal is to generate data that matches the statistical qualities and relationships in the original data while avoiding revealing individual identities or sensitive details.
When you use this artificially generated data, you benefit from not dealing with the limits of using regulated or sensitive data. You can customize the data to fulfill specific requirements that would be impossible to meet with real data. These synthetic data sets are mostly used for quality assurance and software testing.
However, you should be aware that this data also has downsides. Replicating the complexity of the original data may result in discrepancies. It should be noted that this artificially generated data cannot completely replace genuine data, as reliable data is still required to create relevant findings.
Why Use Synthetic Data?
When it comes to data analysis and machine learning, synthetic data provides several advantages that make it a vital tool in your toolbox. By creating data that reflects the statistical features of real-world data, you can open up new opportunities while maintaining privacy, cooperation, and the development of robust models.
Privacy Concerns
Assume you’re working with sensitive data, such as medical records, personal identifiers, or financial information. Synthetic data will act as a shield, allowing you to extract useful insights without exposing individuals’ privacy.
You can maintain confidentiality while conducting critical analysis by generating statistically similar data that is not identifiable to real people.
Data Sharing and Collaboration
This artificially generated data shines as a solution in situations when data exchange presents challenges like legal limits, proprietary issues, or cross-border legislation.
Using synthetically generated datasets, you may stimulate collaboration without revealing sensitive information. Researchers, institutions, and companies can exchange vital knowledge without the typical restrictions.
Model Development and Testing
You can develop accurate, efficient models with synthetically generated data. Consider it your testing space. You may effectively fine-tune your models by testing them on carefully prepared synthetic test data that replicates real-world distributions.
This artificial data will help you detect problems early. It prevents overfitting and ensures the accuracy of your models before deploying them in real-world scenarios.
Types of Synthetic Data
Synthetic data offers many methods to suit your needs. These techniques protect sensitive data while retaining important statistical insights from your original data. Synthetic data can be divided into three types, each with its own purpose and benefits:
1. Fully Synthetic Data
This artificial data is entirely made up and contains no original information. In this scenario, as the data generator, you would normally estimate the density function parameters of the features present in the real data. Then, using the projected density functions as a guide, privacy-protected sequences are created randomly for each characteristic.
Let’s say you decide to replace a small number of real data attributes with artificial ones. The protected sequences for these features align with the other properties found in the actual data. Because of this alignment, the protected and real sequences can be ranked similarly.
2. Partially Synthetic Data
This artificial data comes into play when it comes to protecting privacy while keeping the integrity of your data. Here, selected sensitive feature values that offer a high risk of disclosure are replaced with synthetic alternatives.
To create this data, approaches like multiple imputation and model-based methods are used. These methods can also be used to impute missing values from your actual data. The goal is to keep your data’s structure intact while preserving your privacy.
3. Hybrid Synthetic Data
This artificial data emerges as a formidable alternative for achieving a well-balanced compromise between privacy and utility. A hybrid dataset is created by mixing actual and artificially created data aspects.
A closely related record from the synthetic data vault is chosen for each random record in your real data. This method combines the advantages of totally synthetic and partially artificial data, finding a compromise between excellent privacy preservation and data value.
However, because of the combination of real and synthetic elements, this method can require more memory and processing time.
Synthetic Data Generation Methods
You can explore a range of synthetic data-generating methods, each offering an individual technique for producing data that accurately reflects the complexities of the actual world.
These techniques allow you to produce datasets that preserve the statistical foundations of real data while opening up fresh possibilities for exploration. Let’s explore these approaches:
Statistical Distribution
In this method, you draw numbers from the distribution by studying real statistical distributions and reproducing similar data. When real data is unavailable, you can use this factual data.
Data scientists can construct a random dataset if they understand real data’s statistical distribution. Normal, chi-square, exponential, and other distributions can do this. The accuracy of the trained model is strongly dependent on the data scientist’s expertise with this method.
Agent-Based Modeling
This method allows you to design a model that will explain observed behavior and will produce random data using the same model. This is the process of fitting actual data to a known data distribution. This technology can be used by businesses to generate synthetic data.
Other machine-learning approaches can also be employed to customize the distributions. However, when the data scientists wish to forecast the future, the decision tree will overfit due to its simplicity and ascending to full depth.
Generative Adversarial Networks (GANs)
In this generative model, two neural networks collaborate to generate manufactured, but possibly valid, data points. One of these neural networks acts as a creator, generating synthetic data points. On the other hand, the other network serves as a judge, learning how to differentiate between created fake samples and actual ones.
GANs may be challenging to train and computationally expensive, but the return is well worth it. With GANs, you can generate data that accurately reflects reality.
Variational Autoencoders (VAEs)
It’s a method without supervision that can learn the distribution of your original dataset. It can generate artificial data via a two-step transformation process known as an encoded-decoded architecture.
The VAE model produces a reconstruction error, which can be reduced through iterative training sessions. By using VAE, you can obtain a tool that allows you to generate data that closely resembles the distribution of your real dataset.
Challenges and Considerations
When dealing with synthetic data, be prepared to face several challenges and limits that can have an impact on its effectiveness and applicability:
- Accuracy of Data Distribution: Replicating the precise distribution of real-world data can be difficult, potentially leading to mistakes in generated artificial data.
- Maintaining Correlations: It is difficult to maintain complicated correlations and dependencies between variables, which impacts the reliability of the synthetic data.
- Generalization to Real Data: Models trained on artificial data may not perform as well as expected on real-world data, needing thorough validation.
- Privacy vs. Utility: Finding an acceptable balance between privacy protection and data utility can be difficult, as severe anonymization can compromise the data’s representativeness.
- Validation and Quality Assurance: Because there is no ground truth, thorough validation procedures are required to ensure the quality and dependability of synthetic information.
- Ethical and legal considerations: Mishandling artificial data can raise ethical problems and legal consequences, which highlights the importance of suitable usage agreements.
Validation and Evaluation
When working with artificial data, thorough validation and evaluation are required to ensure its quality, applicability, and reliability. Here’s how to effectively validate and evaluate this fake data:
Measuring Data Quality
- Comparing Descriptive Statistics: To verify alignment, compare the statistical attributes of this artificial data to real data (e.g., mean, variance, distribution).
- Visual Inspection: Visually identify discrepancies and variances by plotting synthetic data against real data.
- Outlier Detection: Look for outliers that could impact artificial data quality and model performance.
Ensuring Utility and Validity
- Alignment of Use Cases: Determine whether the artificial data meets the requirements of your specific use case or research issue.
- Model Impact: Train machine learning models and then evaluate their value on real data.
- Domain Expertise: Include domain experts in the validation process to ensure that the artificial data captures essential domain-specific properties.
Benchmarking Synthetic Data
- Comparison to Ground Truth: If accessible, compare generated data to ground truth data to determine its accuracy.
- Model Performance: Compare the performance of machine learning models trained on synthetic data against models trained on real data.
- Sensitivity Analysis: Determine the sensitivity of results to changes in data parameters and creation methods.
Continuous Development
- Feedback Loop: Continuously improve and adjust data depending on validation and evaluation feedback.
- Incremental Changes: Adjust generation processes gradually to increase data quality and alignment.
Real-World Use Cases
Synthetic data finds application in a diverse range of real-world scenarios, offering solutions to various challenges across different domains. Here are some notable use cases where the artificial data proves its value:
- Healthcare and Medical Research: Synthetic data in healthcare and medical studies is used to distribute and evaluate medical data without compromising patient privacy. Simulating patient records, medical imaging, and genetic data allows researchers to create and test algorithms without exposing sensitive data.
- Financial Analysis: This artificial data tests investment strategies, risk management models, and trading algorithms. Analysts can test alternative scenarios and make informed conclusions. They can do so without using sensitive financial data by recreating market behaviors and financial data.
- Fraud detection: Without revealing client data, financial institutions can develop synthetic transaction data that simulates fraud. This helps develop and improve fraud detection systems.
- Social Sciences: Without breaching privacy, social scientists can analyze trends, habits, and social interactions. Researchers can examine and model human behavior, perform surveys, and simulate social settings to understand societal dynamics.
- Online Privacy Protection: Fake data can preserve consumers’ privacy in privacy-sensitive applications like online advertising or customized recommendation systems. Advertisers and platforms can optimize ad targeting and user experiences using synthetic user profiles and behaviors to maintain user anonymity.
Future Trends in Synthetic Data
As you look ahead, several exciting trends are shaping the future of synthetic data, impacting how you generate and use data for various purposes:
- Customization for Your Needs: In the future, technologies will be available. These will let you customize synthetic data to particular industries or your own needs, and this customization will increase relevance.
- Federated Learning and Privacy Focus: The artificial data will be used with federated learning strategies. These strategies will employ differential privacy to secure data privacy while cooperatively training models.
- The Rise of Data Augmentation: Synthetic information will progressively complement real datasets through data augmentation. This will improve model resilience and performance.
- Ethical and bias considerations: Tools for detecting and mitigating biases will emerge, which will support fairness in AI applications.
- Standardization and Transparency: To improve trustworthiness and openness, it’s important to look out for initiatives aimed at standardizing the data methods. Additionally, look for efforts to develop benchmark datasets.
- Transfer Learning Integration: Synthetic information may be crucial in pretraining models on simulated data. This can decrease the need for large real data for certain tasks.
Conclusion
The potential of synthetic data is becoming clearer. By strategically adding it to your toolkit, you can empower yourself to face obstacles creatively and precisely.
Data scientists can utilize synthetic data to its maximum potential. Their expertise can lead the way for data privacy protection. It can also enrich model development with diverse and adaptable datasets and foster collaboration that transcends conventional boundaries.
QuestionPro can be a significant resource in realizing the possibilities of synthetic data. The platform empowers you to take full advantage of the benefits of synthetic data for your research, analysis, and decision-making processes with our extensive range of tools and features.
Use QuestionPro’s survey design software to collect accurate data from your target audience. This genuine data serves as the foundation for producing significant fake data. You can use QuestionPro to convert raw survey responses into structured datasets. This results in a smooth transition from raw data to synthesized information.
With the help of QuestionPro’s complete tools and experience, you can confidently enter the future of data science.