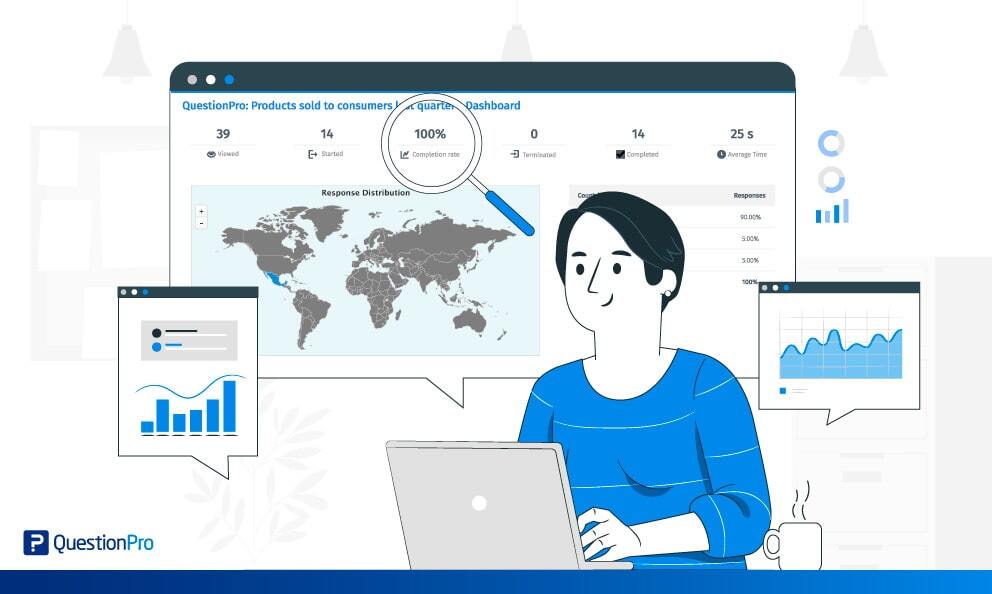
Nowadays, data is collected at various stages of processes and transactions, which has the potential to improve the way we work significantly. However, to fully realize the value of data analysis, this data must be analyzed to gain valuable insights into improving products and services.
Data analysis consists aspect of making informed decisions in various industries. With the advancement of technology, it has become a dynamic and exciting field But what is it in simple words?
What is Data Analysis?
Data analysis is the science of examining data to conclude the information to make decisions or expand knowledge on various subjects. It consists of subjecting data to operations. This process happens to obtain precise conclusions to help us achieve our goals, such as operations that cannot be previously defined since data collection may reveal specific difficulties.
“A lot of this [data analysis] will help humans work smarter and faster because we have data on everything that happens.” –Daniel Burrus, business consultant and speaker on business and innovation issues.
Why is data analytics important?
Data analytics help businesses understand the target market faster, increase sales, reduce costs, increase revenue, and allow for better problem-solving. Data analysis is important for several reasons, as it plays a critical role in various aspects of modern businesses and organizations. Here are some key reasons why data analysis important is crucial:
Informed decision-making
Data analytics helps businesses make more informed and data-driven decisions. By analyzing data, organizations can gain insights into customer behavior, market trends, and operational performance, enabling them to make better choices that are supported by evidence rather than relying on intuition alone.
Identifying opportunities and challenges
Data analytics allows businesses to identify new opportunities for growth, product development, or market expansion. It also helps identify potential challenges and risks, allowing organizations to address them proactively.
Improving efficiency and productivity
Organizations can identify inefficiencies and bottlenecks by analyzing processes and performance data, leading to process optimization and improved productivity. This, in turn, can result in cost savings and better resource allocation.
Customer understanding and personalization
Data analytics enables businesses to understand their customers better, including their preferences, buying behaviors, and pain points. With this understanding, organizations can offer personalized products and services, enhancing customer satisfaction and loyalty.
Competitive advantage
Organizations that leverage data analytics effectively gain a competitive edge in today’s data-driven world. By analyzing data, businesses can identify unique insights and trends that better understand the market and their competitors, helping them stay ahead of the competition.
Performance tracking and evaluation
Data analytics allows organizations to track and measure their performance against key performance indicators (KPIs) and goals. This helps in evaluating the success of various strategies and initiatives, enabling continuous improvement.
Predictive analytics
Data analytics can be used for predictive modeling, helping organizations forecast future trends and outcomes. This is valuable for financial planning, demand forecasting, risk management, and proactive decision-making.
Data-driven innovation
Data analytics can fuel innovation by providing insights that lead to the development of new products, services, or business models. Innovations based on data analysis can lead to groundbreaking advancements and disruption in various industries.
Fraud detection and security
Data analytics can be used to detect anomalies and patterns indicative of fraudulent activities. It plays a crucial role in enhancing security and protecting businesses from financial losses and reputational risk.
Regulatory compliance
In many industries, regulations, and laws are mandatory. Data analytics can help organizations ensure that they meet these compliance requirements by tracking and auditing relevant data.
Types of data analysis
There are several types of data analysis, each with a specific purpose and method. Let’s talk about some significant types:
Descriptive Analysis
Descriptive analysis is used to summarize and describe the main features of a dataset. It involves calculating measures of central tendency and dispersion to describe the data. The descriptive analysis provides a comprehensive overview of the data and insights into its properties and structure.
LEARN ABOUT: Descriptive Analysis
Inferential Analysis
The inferential analysis is used statistical analysis plan and testing to make inferences about the population parameters, such as the mean or proportion. This unit of analysis involves using models and hypothesis testing to make predictions and draw conclusions about the population.
LEARN ABOUT: Statistical Analysis Methods
Predictive Analysis
Predictive analysis is used to predict future events or outcomes based on historical data and other relevant information. It involves using statistical models and machine learning algorithms to identify patterns in the data and make predictions about future outcomes.
Prescriptive Analysis
Prescriptive analysis is a decision-making analysis that uses mathematical modeling, optimization algorithms, and other data-driven techniques to identify the action for a given problem or situation. It combines mathematical models, data, and business constraints to find the best move or decision.
Text Analysis
Text analysis is a process of extracting meaningful information from unstructured text data. It involves a variety of techniques, including natural language processing (NLP), text mining, sentiment analysis, and topic modeling, to uncover insights and patterns in text data.
Diagnostic Analysis
The diagnostic analysis seeks to identify the root causes of specific events or outcomes. It is often used in troubleshooting problems or investigating anomalies in data.
LEARN ABOUT: Data Analytics Projects
Uses of data analysis
It is used in many industries regardless of the branch. It gives us the basis for making decisions or confirming a hypothesis.
Marketing
A researcher or data analyst mainly performs data analysis to predict consumer behavior and help companies place their products and services in the market accordingly. For instance, sales data analysis can help you identify the product range not-so-popular in a specific demographic group. It can give you insights into tweaking your current marketing campaign to better connect with the target audience and address their needs.
Human Resources
Organizations can use data analysis tools to offer a great experience to their employees and ensure an excellent work environment. They can also utilize the data to find out the best resources whose skill set matches the organizational goals.
Academics
Universities and academic institutions can perform the analysis to measure student performance and gather insights on how certain behaviors can further improve education.
Techniques for data analysis
It is essential to analyze raw data to understand it. We must resort to various data analysis techniques that depend on the type of information collected, so it is crucial to define the method before implementing it.
- Qualitative data: Researchers collect qualitative data from the underlying emotions, body language, and expressions. Its foundation is the data interpretation of verbal responses. The most common ways of obtaining this information are through open-ended interviews, focus groups, and observation groups, where researchers generally analyze patterns in observations throughout the data collection phase.
- Quantitative data: Quantitative data presents itself in numerical form. It focuses on tangible results.
Data analysis focuses on reaching a conclusion based solely on the researcher’s current knowledge. How you collect your data should relate to how you plan to analyze and use it. You also need to collect accurate and trustworthy information.
Many data collection techniques exist, but experts’ most commonly used method is online surveys. It offers significant benefits, such as reducing time and money compared to traditional data collection methods.
Data analysis and data analytics are two interconnected but distinct processes in data science. Data analysis involves examining raw data using various techniques to uncover patterns, correlations, and insights. It’s about understanding historical data to make informed conclusions. On the other hand, data analytics goes a step further by utilizing those insights to predict future trends, prescribe actions, and guide decision-making.
At QuestionPro, we have an accurate tool that will help you professionally make better decisions.
Data Analysis Methods
The term data analysis technique has often been used interchangeably by professional researchers. Frequently people also throw out the previous analysis type. We’re hoping for this to be an important distinction between how and when data analyses are done.
However, there are many different techniques that allow for data analysis. Here are some of the main common methods used for data analysis:
Descriptive Statistics
Descriptive statistics involves summarizing and describing the main features of a dataset, such as mean, median, mode, standard deviation, range, and percentiles. It provides a basic understanding of the data’s distribution and characteristics.
Inferential Statistics
Inferential statistics are used to make inferences and draw conclusions about a larger population based on a sample of data. It includes techniques like hypothesis testing, confidence intervals, and regression analysis.
Data Visualization
Data visualization is the graphical representation of data to help analysts and stakeholders understand patterns, trends, and insights. Common visualization techniques include bar charts, line graphs, scatter plots, heat maps, and pie charts.
Exploratory Data Analysis (EDA)
EDA involves analyzing and visualizing data to discover patterns, relationships, and potential outliers. It helps in gaining insights into the data before formal statistical testing.
Predictive Modeling
Predictive modeling uses algorithms and statistical techniques to build models that can make predictions about future outcomes based on historical data. Machine learning algorithms, such as decision trees, logistic regression, and neural networks, are commonly used for predictive modeling.
Time Series Analysis
Time series analysis is used to analyze data collected over time, such as stock prices, temperature readings, or sales data. It involves identifying trends and seasonality and forecasting future values.
Cluster Analysis
Cluster analysis is used to group similar data points together based on certain features or characteristics. It helps in identifying patterns and segmenting data into meaningful clusters.
Factor Analysis and Principal Component Analysis (PCA)
These techniques are used to reduce the dimensionality of data and identify underlying factors or components that explain the variance in the data.
Text Mining and Natural Language Processing (NLP)
Text mining and NLP techniques are used to analyze and extract information from unstructured text data, such as social media posts, customer reviews, or survey responses.
Qualitative Data Analysis
Qualitative data analysis involves interpreting non-numeric data, such as text, images, audio, or video. Techniques like content analysis, thematic analysis, and grounded theory are used to analyze qualitative data.
Quantitative Data Analysis
Quantitative analysis focuses on analyzing numerical data to discover relationships, trends, and patterns. This analysis often involves statistical methods.
Data Mining
Data mining involves discovering patterns, relationships, or insights from large datasets using various algorithms and techniques.
Regression Analysis
Regression analysis is used to model the relationship between a dependent variable and one or more independent variables. It helps understand how changes in one variable impact the other(s).
Step-by-step guide data analysis
With these five steps in your data analysis process, you will make better decisions for your business because data that has been well collected and analyzed support your choices.
LEARN ABOUT: Data Mining Techniques
Step 1: Define your questions
Start by selecting the right questions. Questions should be measurable, clear, and concise. Design your questions to qualify or disqualify possible solutions to your specific problem.
Step 2: Establish measurement priorities
This step divides into two sub-steps:
- Decide what to measure: Analyze what kind of data you need.
- Decide how to measure it: Thinking about how to measure your data is just as important, especially before the data collection phase, because your measurement process supports or discredits your thematic analysis later on.
Step 3: Collect data
With the question clearly defined and your measurement priorities established, now it’s time to collect your data. As you manage and organize your data, remember to keep these essential points in mind:
- Before collecting new data, determine what information you could gather from existing databases or sources.
- Determine a storage and file naming system to help all team members collaborate in advance. This process saves time and prevents team members from collecting the same information twice.
- If you need to collect data through surveys, observation, or interviews, develop a questionnaire in advance to ensure consistency and save time.
- Keep the collected data organized with a log of collection dates, and add any source notes as you go along.
Step 4: Analyze the data
Once you’ve collected the correct data to answer your Step 1 question, it’s time to conduct a deeper statistical analysis. Find relationships, identify trends, and sort and filter your data according to variables. You will find the exact data you need as you analyze the data.
Step 5: Interpret the results
After analyzing the data and possibly conducting further research, it is finally time to interpret the results. Ask yourself these key questions:
- Does the data answer your original question? How?
- Does the data help you defend any objections? How?
- Are there any limitations to the conclusions, any angles you haven’t considered?
If the interpretation of data holds up under these questions and considerations, you have reached a productive conclusion. The only remaining step is to use the process results to decide how you will act.
Join us as we look into the most frequently used question types and how to analyze your findings effectively.
Make the right decisions by analyzing data the right way!
Data analysis advantages
Many industries use data to draw conclusions and decide on actions to implement. It is worth mentioning that science also uses to test or discard existing theories or models.
There’s more than one advantage to data analysis done right. Here are some examples:
- Make faster and more informed business decisions backed by facts.
- Identify performance issues that require action.
- Gain a deeper understanding of customer requirements, which creates better business relationships.
- Increase awareness of risks to implement preventive measures.
- Visualize different dimensions of the data.
- Gain competitive advantage.
- A better understanding of the financial performance of the business.
- Identify ways to reduce costs and thus increase profits.
These questions are examples of different types of data analysis. You can include them in your post-event surveys aimed at your customers:
- Qualitative research analysis focuses on opinions, attitudes, and beliefs.
- Questions start with: Why? How?
Example of qualitative data research analysis: Panels where a discussion is held, and consumers are interviewed about what they like or dislike about the place.
- Quantitative research analysis focuses on complex data and information that can be counted.
- Data is collected by asking questions like: How many? Who? How often? Where?
Example of quantitative research analysis: Surveys focused on measuring sales, trends, reports, or perceptions.
Data analysis with QuestionPro
Data analysis is crucial in aiding organizations and individuals in making informed decisions by comprehensively understanding the data. If you’re in need of various data analysis techniques solutions, consider using QuestionPro. Our software allows you to collect data easily, create real-time reports, and analyze data. Practical business intelligence relies on the synergy between analytics and reporting, where analytics uncovers valuable insights, and reporting communicates these findings to stakeholders.
LEARN ABOUT: Average Order Value
Start a free trial or schedule a demo to see the full potential of our powerful tool. We’re here to help you every step of the way!